If you’ve ever done any sort of serious training or racing as a cyclist, you’ll know all about Functional Threshold Power (FTP). You’ll know that it’s an estimation of the highest power you can maintain for an hour (or thereabouts), and you’ll know that it can be used to help guide your training. You probably also know about the ‘95% Rule’ – the idea that you can estimate your FTP by doing a maximum 20-minute effort and taking 95% of your average power from that effort.
Well, new research seems to be adding further weight to claims that this long-used calculation – the ‘95% Rule’ – is inaccurate … which is kind of a problem for anyone looking to get the most from their training.
Basics of FTP
Before we go any further, let’s go back to basics. FTP is defined as “the highest power that a rider can maintain in a quasi-steady state without fatiguing for approximately one hour.” On its own, this number is not particularly useful. What it can be used for, though, is determining training zones, to maximise the impact of your training.
Spending time in particular zones, for particular amounts of time, can help target your training in the most effective way. While there are different ways of slicing power zones, the most commonly used divisions look like this:
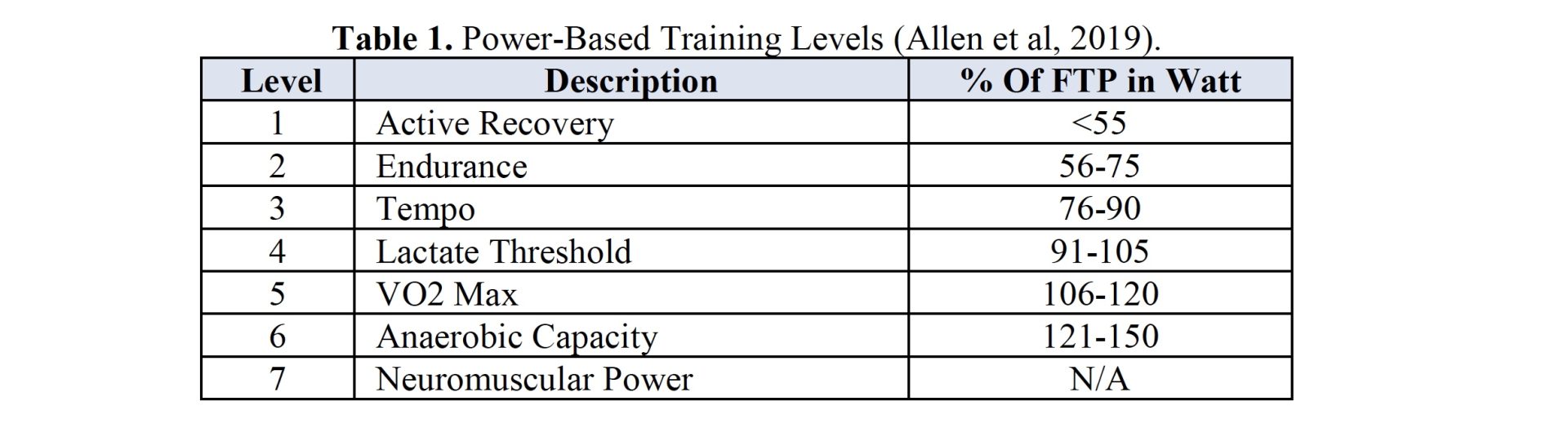
There are other ways of working out these training zones. For example, lab testing can be used to determine your lactate threshold – the point at which lactate starts accumulating in your blood faster than your body can flush it out. This threshold happens at a certain power output and a rider’s training zones can be built out from there.
But FTP is an easier route to take. You don’t need to go into a lab to do the testing – you just need a power meter and a willingness to suffer. And so FTP has become the preferred option for most cyclists.
For years there’s been a common method for determining a rider’s FTP. Sure, in theory you could just go out and ride an hour as hard as you can but that’s incredibly taxing, it can interfere with other training or racing efforts, and for many cyclists, holding a hard but steady effort for an hour is just not possible. Instead, most cyclists use the simple estimation mentioned above: the 95% Rule. Do a 20-minute max effort*, take 95% of your average power and use that as an estimate of FTP.
Of course, if your training zones are going to be based on that FTP figure, it’s important that your FTP is accurate. If your FTP estimate is too high, you’re likely to train at higher intensities than is ideal. If it’s too low, you’re likely not training as hard as you could or should be.
For a few years now some people have been saying that the 95% Rule isn’t as accurate as first thought. And now, a couple of researchers have added weight to that argument. They claim there’s a better, more accurate way of determining FTP.
(*If you follow the most commonly used protocol, created by Andy Coggan and Hunter Allen, you're supposed to do a five-minute max effort first, and then do your 20-minute effort.)
Is the 95% Rule accurate?
This latest paper, presented at the International Conference on Intelligent Data Engineering and Automated Learning, comes from two researchers: Andrew Stockwell, a data scientist and coach; and Andrea Corradini a senior lecturer in digital transformation at the MCI University in Austria.
The pair say that while there have been previous attempts to validate the 95% Rule (what they call “estimated FTP” or “eFTP”), the sample sizes used in previous studies were small, or those studies were constrained to (usually male) riders of above-average strength. Stockwell and Corradini’s approach was to take a massive dataset from a whole swag of male and female riders, of all different cycling abilities, and use that data to confirm whether the 95% Rule is accurate across the board. They would then use machine learning – a type of AI – to see if they could come up with a better way of estimating FTP.
The pair pulled their data from an open-source dataset containing some 1.26 million workouts from thousands of different riders (from the Golden Cheetah training platform). The researchers sorted through the data, found each rider’s best power figures for various durations (plus some other stats), then cleaned the data to remove any anomalies. For example, any stats higher than the established limits of human capabilities were excluded. Specifically: “Data with 5-second power above 25.18 watts/kg are excluded,” the researchers write. “Similarly, we excluded 1-minute power data above 11.5 watts/kg, 5-minute power data above 7.6 watts/kg, and 60-minute power data above 6.6 watts/kg.”
They then narrowed the data to riders of ages 16-100, with weights between 50 kg and 150 kg, and ensured that only riders with at least 30 logged rides were included.
With all that done, they crunched the remaining data to see whether 95% of each rider’s 20-minute best lined up with their one-hour best. They found that while the two measures – estimated FTP (eFTP) and actual FTP (aFTP) lined up reasonably well, they were far from a perfect match. They found that the 95% Rule tended to overestimate a rider’s actual FTP.
As they write in their paper: “Most of the data points on this visualization lie below the diagonal line, especially as wattage increases. This means that eFTP, for the most part, is outputting a higher value than is seen in the actual observed FTP.”
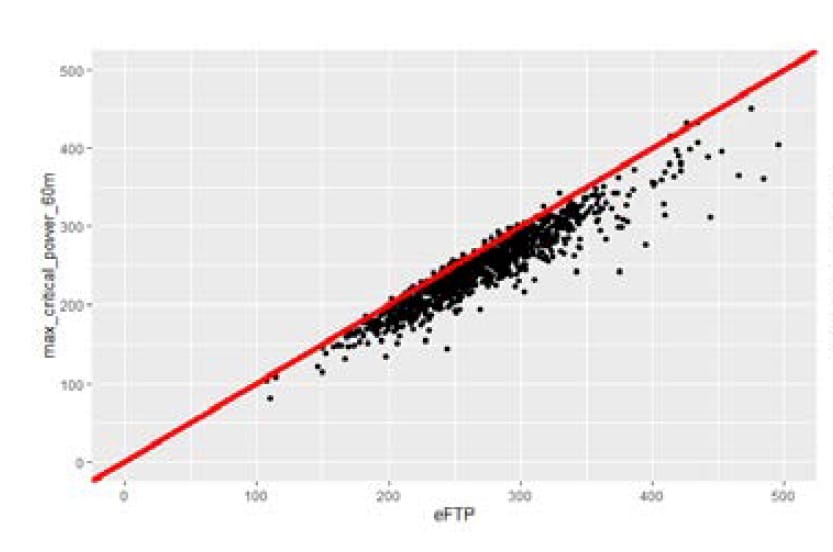
Other studies have found that the 95% Rule overestimates FTP as well. Like this one, published in the International Journal of Physiology and Performance in 2019, which found that taking 90% of a rider’s 20-minute average power would produce a more accurate figure than 95%.
Stockwell and Corradini’s number-crunching suggests that a better value might be something like 86%*. But that’s just the first part of their study. Next they set out to find a better equation that could predict FTP without requiring a rider to do a full 60-minute effort.
(*This was based on the mean value of aFTP (247.39 W) in the dataset, and the mean value for best 20-minute effort (285.22 W).)
Is there a better way?
To create an equation for determining FTP, the researchers took 70% of the data from their dataset and used that to train their AI model. The remaining 30% would be used for testing.
Stockwell and Corradini spend two pages of their paper explaining the “weighted linear regression” used by their machine-learning software to create an equation for FTP. While most of it is well beyond the scope of this discussion, the lay version goes something like this.
The AI looks at all the data in the dataset and tries to work out which variables (e.g. 20-minute power, 1-minute power) are most significant in predicting a rider’s FTP. Then the researchers assess the AI’s output to determine which variables they want to keep in or leave out of the final model. They then run the model again using only the variables they want, repeating that process until they arrive at the final equation.
Here is what that rather-gnarly final equation looks like:
Predicted FTP = 1.358652 + (0.276111 x avg_power_all_rides) - (0.002247 x max_critical_power_5s) – (52.347384 x perc_zone3) + (0.789667 x max_critical_power_30m)
All of the variables above are reasonably self-explanatory except perhaps for perc_zone3. This is the percentage of time a rider spends in zone 3 – the “tempo” zone, usually defined as 76-90% of their FTP – across all their data in the dataset. Note the negative signs in front of the max_critical_power_5s and perc_zone3 factors – the higher these numbers, the lower the final FTP.
Stockwell and Corradini then took their equation and pushed the remaining 30% of data through it – ride data from 1,042 cyclists – to determine each rider’s predicted FTP (pFTP). They then compared this pFTP against their eFTP (the output of the 95% Rule) and their aFTP (their actual best 60-minute effort as found in the dataset).
In short, the model is a significant improvement over the 95% Rule. Note that in the graph below right that all the dots are spread around the line rather than all being below it (like they were for eFTP, on the left). This suggests that the model is not routinely over-estimating FTP like the 95% Rule does.
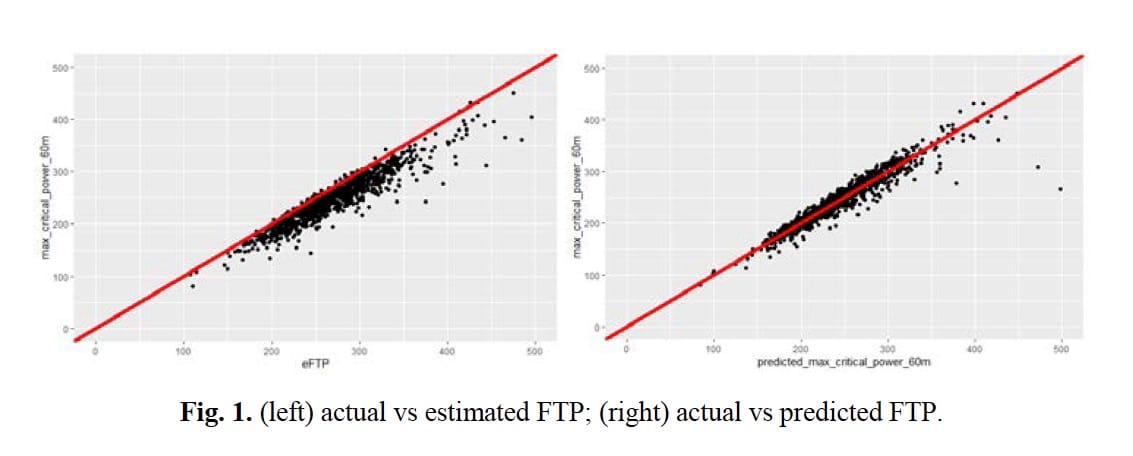
While the prevailing wisdom is that a 20-minute effort is the most useful for determining FTP, Stockwell and Corradini’s model places its emphasis elsewhere. It found that a rider’s best 30-minute effort, and five-second effort were more instructive.
The 30-minute effort, in particular, makes sense – both that and a 60-minute effort are similar, hard endurance efforts. A higher max_critical_power_5s leading to a lower FTP makes some sense too – the best endurance riders usually aren’t the most powerful sprinters. You might note, however, that even though a higher sprint power leads to a lower FTP in the above equation, the difference is minimal. The difference between a max_critical_power_5s of 1,800 watts and 1,250 watts, say, is only a 1.1 W difference to FTP. Almost negligible.
It’s interesting that the AI found time spent in zone 3 to be significant. Like max_critical_power_5s this factor has a minus sign in front of it, which could mean, in the words of the researchers, “cyclists who spend a higher percentage of their training time in this power zone, contribute negatively to their FTP.”
More research is needed to verify this and understand why this might be the case.
Shortcomings
Maybe you’ve already spotted some issues with this study. There are a few.
For starters, remember how Stockwell and Corradini’s model was based on each rider’s best efforts in the dataset? Well, there’s no guarantee each best effort in the dataset was actually the best that rider could do. Sure, a decent percentage of riders will have done a max effort of 20 minutes or 30 minutes, and maybe some have even done an hour full-gas, but each rider’s “actual FTP” is being pulled from the dataset and if they’ve never tried their absolute hardest for that period of time, the data will skew the end result.
There’s a more fundamental question to consider too. This study more or less assumes that a rider’s FTP and best one-hour power are the same thing – indeed the researchers take each rider’s best 60-minute effort from the dataset to be their “actual FTP”. In the words of Escape’s resident training and performance guru, Ronan Mc Laughlin, “in reality, very few people can hold their FTP (regardless of method for calculating) for one hour.”
There’s another type of variance in the dataset that’s potentially problematic. “In a 10-second sprint, an elite cyclist may be able to maintain more than 1,500 W, whereas a recreational cyclist may only be able to maintain 500 W or less,” the researchers write. This, too, is a problem in a model where a short effort of just a few seconds was identified as significant in calculating FTP.
The solution? “Building out future models that are based off a cyclist's current level, could yield more realistic results,” the researchers write. “This would mean running a model for recreational cyclists and a separate one for elite cyclists, therefore minimizing the variance and range of some of the power metrics.”
There were other, less-significant issues too. Power meter accuracy and calibration weren’t accounted for (the best power-related studies have all riders using the same power meter, all calibrated in the same way). Then there’s the fact that “not enough female cyclists [were] included in the dataset. The final model might therefore be skewed to male cyclists.”
And finally, there’s also the issue of practicality. Even if that gnarly equation the researchers came up with is correct, it’s not exactly easy to use.
“The variables max_critical_power_5s and max_critical_power_30m are easy to obtain, however perc_zone3 and avg_power_all_rides require summarization from all power files, or a subset for a defined period,” the researchers admit. “This is something that cannot be completed without a tool that creates these data summaries for the athlete or coach.”
As Stockwell and Corradini note, though, there is a solution to that problem. “If our model was transferred into an online tool that already stored all of a cyclists' power files,” they write, “it could be run every time a new power file was uploaded to calculate a dynamically changing modeled FTP from current and historical data.”
Issues aside, there’s promise to this study. Even if the model isn’t quite right, “the methodology for creating our model still stands true,” the researchers write, “and can therefore be replicated if this data was available from another source that had more controlled methodologies for data capturing”.
Gauging your fitness
In the meantime, if you’re a rider looking to get a baseline FTP reading, to help with your training, you’ve got a few options. The best option probably depends on how much training you’ve been doing recently.
If you haven’t done all that much, you could consider a ramp test. Available on all major indoor training platforms (e.g. Zwift, TrainerRoad etc.) a ramp test starts easy before increasing in intensity every minute until you simply can’t continue. Take 75% of the best one-minute power you managed before exhaustion and that can be used as an estimate of your FTP.
If you’re reasonably fit though, doing a 20-minute test is still a pretty good option. Just don’t use that 95% conversion rate.
“I think it does make sense to drop that 95% to somewhere between 85%-95%,” Stockwell tells me. “The more hours you’re putting in the closer that number will be to 95% though.”
Did we do a good job with this story?